Deep Learning in Healthcare: Revolutionizing Diagnosis and Treatment Prediction
This article explores the transformative role of deep learning in healthcare, showcasing how it's revolutionizing diagnosis and treatment prediction. It highlights key advancements, challenges, and the future potential of integrating AI in medical practices, promising a new era of precision medicine.
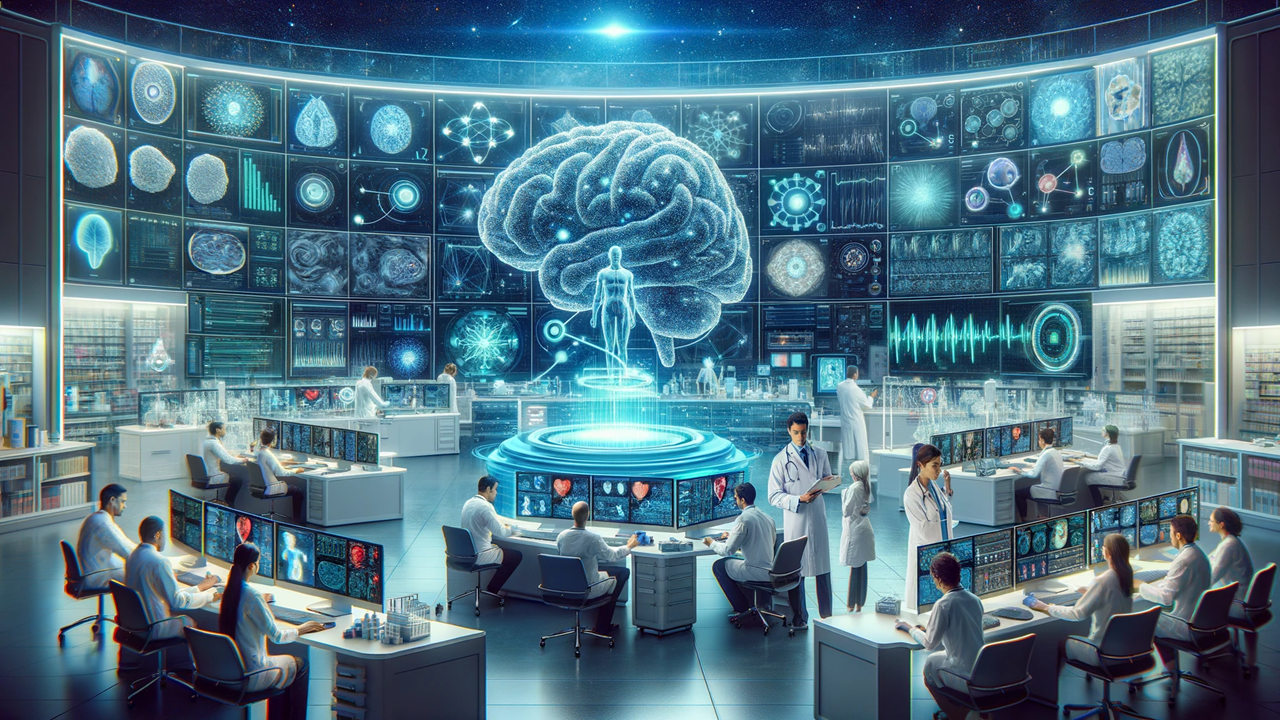
In the ever-evolving landscape of healthcare, the advent of deep learning technologies marks a pivotal shift towards more accurate, efficient, and personalized medical care. This subset of artificial intelligence (AI) is not just enhancing existing procedures; it's fundamentally revolutionizing how diagnoses are made and treatments are predicted, heralding a new era of precision medicine that was once the realm of science fiction.
The Dawn of Deep Learning in Healthcare
Deep learning, a sophisticated form of machine learning, utilizes algorithms known as neural networks, which are inspired by the structure and function of the human brain. These networks can learn from vast amounts of data, identify patterns, and make decisions with minimal human intervention. In healthcare, this capability opens up unprecedented opportunities for early disease detection, accurate diagnosis, and the customization of treatment plans to the individual patient's unique needs.
Transforming Diagnosis
One of the most significant impacts of deep learning is on the diagnosis of diseases. Traditional diagnostic methods, while effective, often rely heavily on the expertise of specialists and can be time-consuming and costly. Deep learning algorithms, trained on thousands or even millions of patient records, images, and other data types, can detect subtleties and patterns beyond human recognition.
In fields like radiology and pathology, deep-learning models are already being used to analyze medical images with remarkable accuracy. For instance, AI systems can now identify signs of diseases such as cancer, diabetic retinopathy, and Alzheimer's from scans and pathology slides, sometimes even before symptoms manifest, allowing for earlier intervention and significantly better patient outcomes.
Advancing Treatment Prediction
Deep learning is also making strides in predicting treatment outcomes, enabling healthcare providers to tailor therapies to individual patients. By analyzing vast datasets, AI models can predict how different patients will respond to various treatments based on genetic factors, lifestyle, and other health data. This not only enhances the effectiveness of treatments but also minimizes the risk of adverse reactions, paving the way for truly personalized medicine.
For example, in oncology, deep learning algorithms are used to predict the effectiveness of chemotherapy, targeted therapy, and immunotherapy, based on the genetic makeup of a patient's tumor. This approach not only spares patients from undergoing ineffective treatments but also significantly reduces the time and cost associated with trial-and-error treatment methods.
Overcoming Challenges
Despite its potential, the integration of deep learning into healthcare is not without challenges. Data privacy and security are major concerns, given the sensitive nature of health records. Additionally, there's the issue of data bias—AI systems are only as good as the data they're trained on. If the data is not diverse, the system's recommendations may not apply to all patient demographics.
Moreover, the "black box" nature of deep learning algorithms, where the decision-making process is not always transparent, raises questions about accountability and trust. Bridging this gap requires ongoing efforts to make AI models more interpretable and to ensure that healthcare professionals are adequately trained to work alongside AI tools.
The Future of Deep Learning in Healthcare
Looking forward, the role of deep learning in healthcare is set to expand. Beyond diagnosis and treatment prediction, AI is poised to improve patient monitoring, drug discovery, and health system management. Wearable devices that monitor vital signs and predict health issues before they become serious are already in development. In drug discovery, AI algorithms are accelerating the identification of promising compounds and predicting their effectiveness, drastically reducing the time and cost of bringing new drugs to market.
As AI technologies continue to evolve, so too will their integration into healthcare, driven by the dual engines of innovation and necessity. The COVID-19 pandemic, for instance, has highlighted the potential of AI in tracking disease spread, predicting outbreaks, and analyzing public health data in real time.
Conclusion
Deep learning in healthcare represents a convergence of technology and medicine that is transforming the landscape of diagnosis and treatment prediction. By harnessing the power of AI, healthcare professionals can offer more accurate, efficient, and personalized care, ultimately improving patient outcomes and advancing public health. However, realizing this potential fully requires navigating the challenges of data privacy, bias, and transparency. As we move forward, the collaboration between technologists, healthcare providers, and policymakers will be critical in shaping a future where deep learning technologies are seamlessly integrated into healthcare systems, ensuring they serve the best interests of patients worldwide. In this new era of precision medicine, deep learning stands as a beacon of hope, offering solutions to some of healthcare's most enduring challenges and opening the door to possibilities yet unimagined.
ALSO READ
Graph-CNNpred: Advanced Deep Learning for Superior Accuracy in Stock Market Trend Prediction
Abu Dhabi Names Road After Indian-Origin Doctor for Healthcare Contributions
Ramaiah Memorial Hospital Partners with Mount Sinai for Enhanced Healthcare Excellence
UAE names street after Indian-origin doctor for his 60-year contribution to healthcare
Sri Sathya Sai University and Posspole Unite to Revolutionize Healthcare Innovation