Aerial and AI Technology: Next-Gen Tools for Effective Marine Debris Monitoring
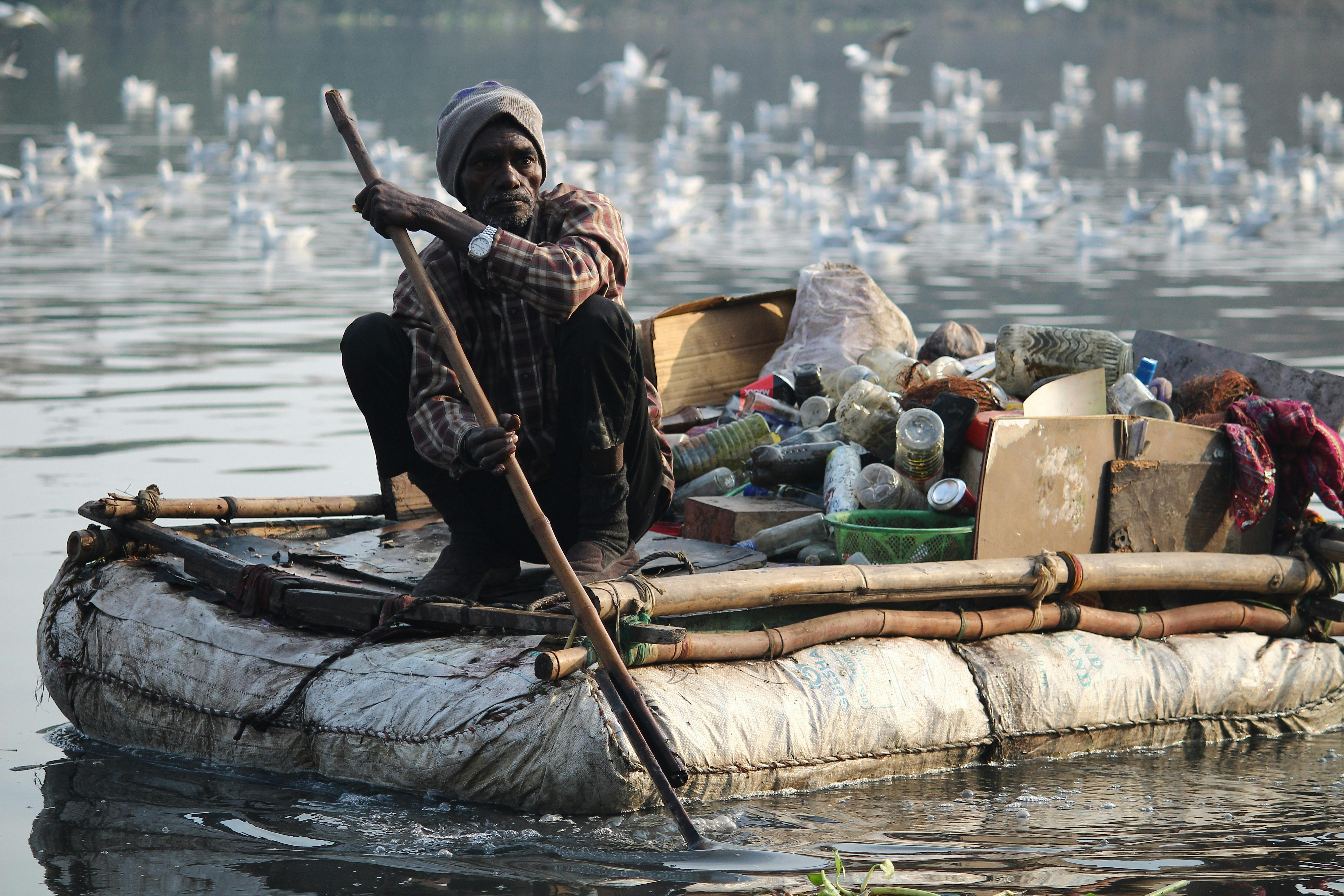
Marine debris is a pervasive issue that impacts the health of marine ecosystems globally. It poses risks to various forms of marine life and contributes to pollution that can have long-lasting effects on the environment. Traditional methods for monitoring and managing marine debris typically involve manual surveys, which are time-consuming, costly, and often impractical for large-scale assessments.
In response to these challenges, researchers from 'Davis Institute for AI, Colby College Waterville, USA, Bigelow Laboratory for Ocean Sciences, USA, Department of Chemistry, Colby College Waterville, USA' have been exploring the use of technology to enhance the efficiency and accuracy of debris monitoring. The integration of drones and advanced computer vision techniques represents a significant step forward in this regard. Drones can cover large areas quickly and capture high-resolution images, while computer vision algorithms can process these images to identify and classify debris.
Framework Overview
The framework introduced in the study combines several state-of-the-art technologies to create an effective solution for marine debris monitoring. Grounding DINO and CLIP are central to this approach, providing robust object detection and classification capabilities without the need for extensive labeled datasets. This zero-shot learning approach is particularly advantageous in scenarios where labeled data is scarce or unavailable.
What is Grounding DINO and CLIP?
Grounding DINO is a transformer-based model that excels in detecting objects in images. It leverages the power of transformers to capture complex patterns and relationships within the data, enabling it to identify debris objects accurately. CLIP model, on the other hand, is a vision-language model that can classify objects based on textual descriptions. By combining these two models, the framework can detect and classify debris objects with high precision.
To address the issue of duplicate detections, the framework incorporates SIFT for duplicate matching. SIFT is a well-established technique in computer vision that identifies key features within an image and matches them across different views. This helps in ensuring that each piece of debris is counted only once, even if it appears in multiple images.
Web Application Development
A significant aspect of the framework is the development of a web application that facilitates the entire process from image capture to analysis and visualization. The application is designed to be user-friendly, allowing community members and researchers to upload drone images, run object detection and classification algorithms, and visualize the results on a map.
The web application supports various functionalities, including:
- Uploading and managing drone images
- Running object detection and classification using the integrated models
- Visualizing detected debris on a map
- Generating reports and summaries of the findings
This application empowers users to conduct debris surveys more efficiently and make informed decisions about cleanup efforts. By providing an accessible platform, the researchers aim to encourage broader participation in marine debris monitoring and management.
Performance Evaluation
The performance of the framework was evaluated across seven debris object classes, demonstrating its effectiveness in real-world scenarios. The mean IoU of 0.69 indicates that the framework can accurately delineate debris objects within images, while the F1 score of 0.74 reflects its ability to classify these objects correctly.
These metrics are comparable to those achieved by supervised methods, which typically require extensive labeled datasets for training. The fact that the framework can perform at this level without labeled data highlights the potential of zero-shot learning approaches in environmental monitoring applications.
Implications and Future Work
The introduction and implication of this framework can have significant impacts on marine debris management. It can reduce the reliance on manual labor and enable automated, scalable surveys, offering a practical solution to a widespread environmental problem. Communities and organizations can adopt this technology to monitor debris accumulation more effectively and coordinate cleanup efforts based on accurate data.
Future work will focus on refining the framework and expanding its capabilities. Potential areas of improvement include enhancing the accuracy of object detection and classification, integrating additional types of data (such as satellite imagery), and developing more sophisticated algorithms for analyzing debris patterns over time.
Furthermore, the researchers plan to collaborate with environmental organizations and government agencies to deploy the framework in various settings. By gathering feedback from these deployments, they aim to continuously improve the system and ensure that it meets the needs of stakeholders involved in marine debris management.
The Future of Marine Debris Monitoring
Marine debris is a critical issue that requires innovative solutions to address effectively. The framework developed in this study represents a significant advancement in the field, combining the power of drones, computer vision, and web technologies to create a comprehensive and efficient debris monitoring system.
By leveraging zero-shot learning techniques, the framework can detect and classify marine debris without the need for extensive labeled datasets, making it a versatile and scalable solution. The accompanying web application provides a user-friendly platform for conducting debris surveys and visualizing the results, empowering communities to take proactive measures in managing marine debris.
The success of this framework in real-world evaluations underscores its potential to transform how marine debris is monitored and managed. As the researchers continue to refine and expand the system, it is poised to become a vital tool in the global effort to protect marine ecosystems and ensure a cleaner, healthier environment for future generations.