Artificial Intelligence: A New Frontier in the Battle Against Antibiotic Resistance
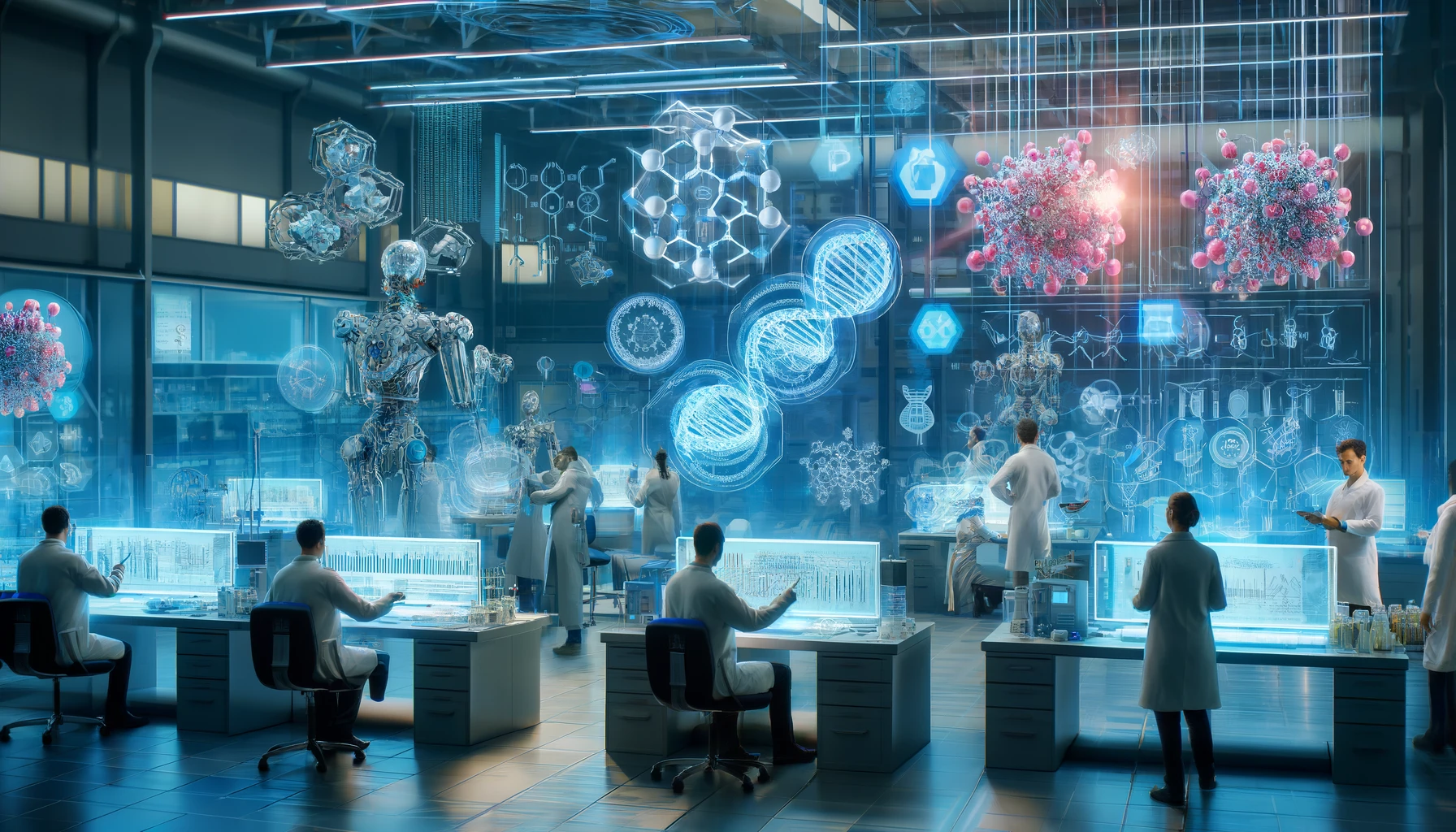
Antibiotic resistance has emerged as a formidable challenge to global public health, driven by the intricate interplay between bacterial genetics and human activity. This crisis is exacerbated by the misuse of antibiotics in both human and veterinary medicine, noncompliance with prescriptions, and extensive use in agriculture, leading to a surge in resistant bacterial strains.
Addressing this issue, researchers from the Campus of Bio-Medico University of Rome and the University of Sassari explored in their comprehensive study that this issue demands innovative solutions and artificial intelligence. AI is at the forefront of this battle, offering groundbreaking approaches to understanding, preventing, and mitigating antibiotic resistance.
AI's Potential in Combating Antibiotic Resistance
AI's potential in combating antibiotic resistance is multifaceted. By analyzing genomic data, AI can swiftly identify resistance markers, facilitating early interventions. This capability is crucial for monitoring and controlling the spread of resistant infections, allowing healthcare providers to act promptly. Additionally, AI-powered decision support systems are revolutionizing antibiotic prescribing practices. These systems analyze vast amounts of patient data, including medical records and microbial profiles, to recommend the most effective antibiotics, reducing the misuse and overprescription that drive resistance.
Harnessing AI for Faster, Cost-Effective Antibiotic Development
The integration of AI in drug discovery is another promising avenue. Machine learning algorithms can sift through extensive chemical databases to identify potential new antibiotics, significantly accelerating the drug development process. This approach not only shortens the time required to bring new drugs to market but also reduces the associated costs. AI can also optimize the design of new molecules, enhancing their efficacy and reducing the likelihood of resistance development.
One notable example is the use of deep learning algorithms to predict the efficacy of new compounds. These models analyze the molecular structures of existing antibiotics and their bacterial targets, suggesting modifications to overcome resistance mechanisms. Furthermore, AI can identify new therapeutic targets by leveraging genomic and transcriptional data, opening up new pathways for treatment.
Despite these advancements, challenges remain. The quality and availability of data are critical for training robust AI models. High-quality, well-annotated datasets are essential to ensure the accuracy and reliability of AI predictions. However, the variability in data quality and the presence of noisy or incomplete data can hamper the performance of these models. Addressing this issue requires concerted efforts to standardize data collection and annotation processes.
Challenges in the Interpretability of AI Models
Another significant challenge is the interpretability of AI models. Many AI algorithms, especially those based on deep learning, operate as black boxes, making it difficult for clinicians to understand the rationale behind their recommendations. Ensuring transparency and interpretability is crucial to gaining the trust of healthcare providers and patients. Techniques such as Local Interpretable Model-agnostic Explanations (LIME) and SHapley Additive exPlanations (SHAP) can help make AI predictions more understandable, promoting their acceptance in clinical settings.
AI's role extends beyond diagnosis and treatment optimization to include the development of novel antibacterial agents. AI-driven virtual screening can identify molecules with potential antibacterial activity, streamlining the discovery process. Generative models can design new molecular structures with desired properties, paving the way for the development of potent and specific antibiotics.
The impact of AI is also evident in optimizing antibiotic use. AI-guided decision support systems can analyze clinical data to suggest the most effective treatments, considering patient history and local resistance patterns. These systems can be continuously updated with new data, improving their recommendations over time. Additionally, AI can aid in the development of personalized antibiotic regimens by analyzing genetic and clinical data to predict individual responses to different antibiotics, tailoring treatments to each patient's unique profile.
However, the successful implementation of AI in healthcare requires addressing regulatory and legal challenges. Establishing clear guidelines for the approval and oversight of AI systems is essential to ensure their safety, efficacy, and privacy. Collaboration among AI developers, healthcare providers, and regulators is crucial to creating a trustworthy environment for the adoption of AI technologies.
AI vs. Superbugs: The Future of Antibiotics
Looking ahead, the integration of AI with other emerging technologies, such as synthetic biology and nanomedicine, holds promise for further advancements in combating antibiotic resistance. AI-based intelligent drug delivery systems can improve the targeting and efficacy of treatments, while predictive modeling can anticipate the emergence of new resistance forms, enabling proactive prevention strategies.
While AI presents significant opportunities in the fight against antibiotic resistance, it is not without challenges. Ensuring high-quality data, transparency, and regulatory compliance are essential for harnessing the full potential of AI in healthcare. With continued innovation and collaboration, AI can play a pivotal role in addressing one of the most pressing public health threats of our time, preserving the efficacy of antibiotics for future generations.
ALSO READ
Zen Technologies Unveils AI-powered Defence Innovations for Global Market
Dell, Qualcomm, and Microsoft Team Up to Launch AI-Powered PCs in India
BIG FM's AI-Powered Initiative 'BIG Dhun' Set to Revolutionize Music Experience
Delhi Courts Launch First AI-Powered Hybrid Courtroom
Nagpur Police Unveil AI-Powered Tool 'SIMBA' to Revolutionize Crime Investigation