Brand-new material system could improve energy efficiency of AI chips
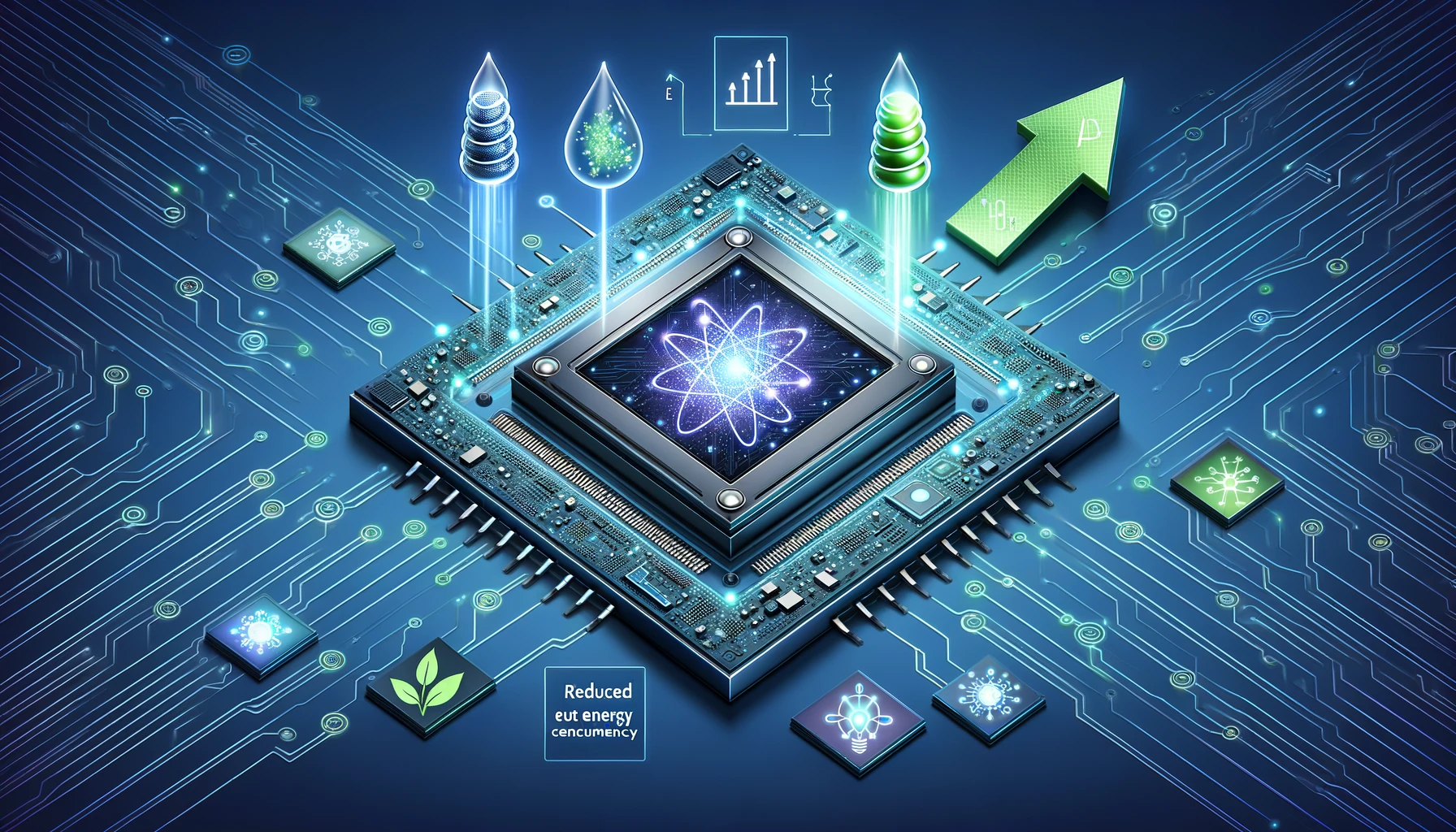
Artificial intelligence (AI) is rapidly transforming our world, but it has a massive energy appetite. With AI projected to consume a staggering 0.5% of global electricity by 2027, researchers are scrambling for solutions that offer the benefits of AI without breaking the power grid).
A new development from the University of Michigan offers a glimmer of hope - memristors (hardware analogues of neurons) with tunable "relaxation times" that could significantly improve the efficiency of AI processing audio and video data.
Memristors, electrical components that store information in their resistance, boast the potential to significantly improve the efficiency of AI processing, particularly when dealing with time-dependent information like audio and video data.
The Bottleneck
Current AI systems rely on graphical processing units (GPUs) that excel at parallel processing, making them ideal for the complex calculations involved in machine learning algorithms. However, this power comes at a steep price - GPUs are notorious for their high energy consumption.
GPUs require constant data movement between internal memory and processing units, which demands significant time and energy, and as the complexity of AI tasks increases, so does the energy footprint.
Memristors: The Game Changer
Memristors offer a fundamentally different approach for powering artificial neural networks. They mimic the way biological and artificial neural networks function, potentially embodying the entire network within themselves.
Memristors could achieve a staggering 90% reduction in energy usage compared to GPUs, making them a highly attractive solution for powering future AI systems.
In biological neural networks, each neuron receives incoming signals from other neurons, but it won't necessarily pass them on. The neuron has a specific window of time to receive enough electrical oomph to fire its own signal. If the signal arrives too late, the neuron "relaxes" and forgets it ever happened. This "relaxation time" is crucial for tasks like recognizing speech or following a video sequence.
Memristors function somewhat differently. Instead of simply detecting the presence or absence of a signal, they modulate how much of the electrical signal passes through. When exposed to a signal, the memristor's resistance decreases, permitting more of the subsequent signal to pass. In memristors, "relaxation" means that the resistance rises again over time.
A brand-new material system
Previously, creating memristors with controlled relaxation times was a challenge. However, scientists have now discovered that tweaking the materials used to build memristors can provide different relaxation times. This is like having different-sized lanes on the highway – some prioritize speed (fast relaxation), while others prioritize holding information for a longer period (slower relaxation).
The researchers used the superconductor YBCO (yttrium, barium, carbon and oxygen) to build their initial memristors. Although YBCO exhibits no electrical resistance at temperatures below -292 degrees Fahrenheit, its crystal structure was the primary interest. This structure helped the team organize the magnesium, cobalt, nickel, copper, and zinc oxides in the memristor material.
By manipulating the ratios of different oxides, the team achieved relaxation times ranging from 159 to 278 nanoseconds (billionths of a second). A simple memristor built with this technology could learn to recognize spoken numbers from zero to nine - even before the entire audio input was complete.
While this process is currently energy-intensive, the team anticipates that a simpler and more scalable method could pave the way for mass production.
"We anticipate that our brand-new material system could improve the energy efficiency of AI chips six times over the state-of-the-art material without varying time constants," stated Sieun Chae, a recent U-M Ph.D. graduate in materials science and engineering and co-first-author of the study with Sangmin Yoo, a recent U-M PhD graduate in electrical and computer engineering.
The study titled "Efficient data processing using tunable entropy-stabilized oxide memristors" is published in the journal Nature Electronics. Wei Lu, the James R. Mellor Professor of Engineering at U-M, is the co-corresponding author of the study with John Heron, U-M associate professor of materials science and engineering.
- READ MORE ON:
- AI
- energy efficient AI chips
- Memristors